3427 videos
Aachen: Storagene - DNA-Based Data Storage (2021) - Project Promotion [English]
Title: Storagene - DNA-Based Data Storage
Description: Humanity faces the challenge of creating large amounts of data every day. We aim to improve the enzymatic DNA-synthesis – utilizing the terminal deoxynucleotidyl transferase (TdT) – t...
Not For Sale - Keeping Your Data Private Through Self-Hosting
Data privacy is important. That's something that everyone should be aware of, but unfortunately, it's not really the case. In a world of free services from companies like Google, Facebook, Microsoft, and others, the allure of giving up your privac...
Annotate to Educate: The Dual Life of a Syrian Student & Data Annotator
This short film highlights the inadequate training of data workers in Syria and their resulting personal struggles. It advocates for fair, structured, and honest training processes to empower and prepare them for the AI industry's demands.
By...
Data Privacy Tech for 2021 (TILvids.com Exclusive)
Well everyone, we've almost done it, another year in the books. And well...it's certainly been a year, hasn't it? The environment has seen better days to be sure, politics in general is messy to say the least, and oh yes, who could forget our love...
Data Interception Environment Part 1: set up
You can find a written version of this video on our website: https://privacyinternational.org/explainer/4717/how-use-data-interception-environment
And detailed documentation on our GitHub: https://github.com/privacyint/ (where you can also downlo...
93 channels
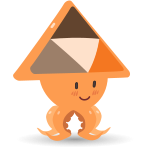
Data_Channel
J'aime les cours de japonais de Julien mais YT pas du tout. Soutenez le sur sa chaine officielle.
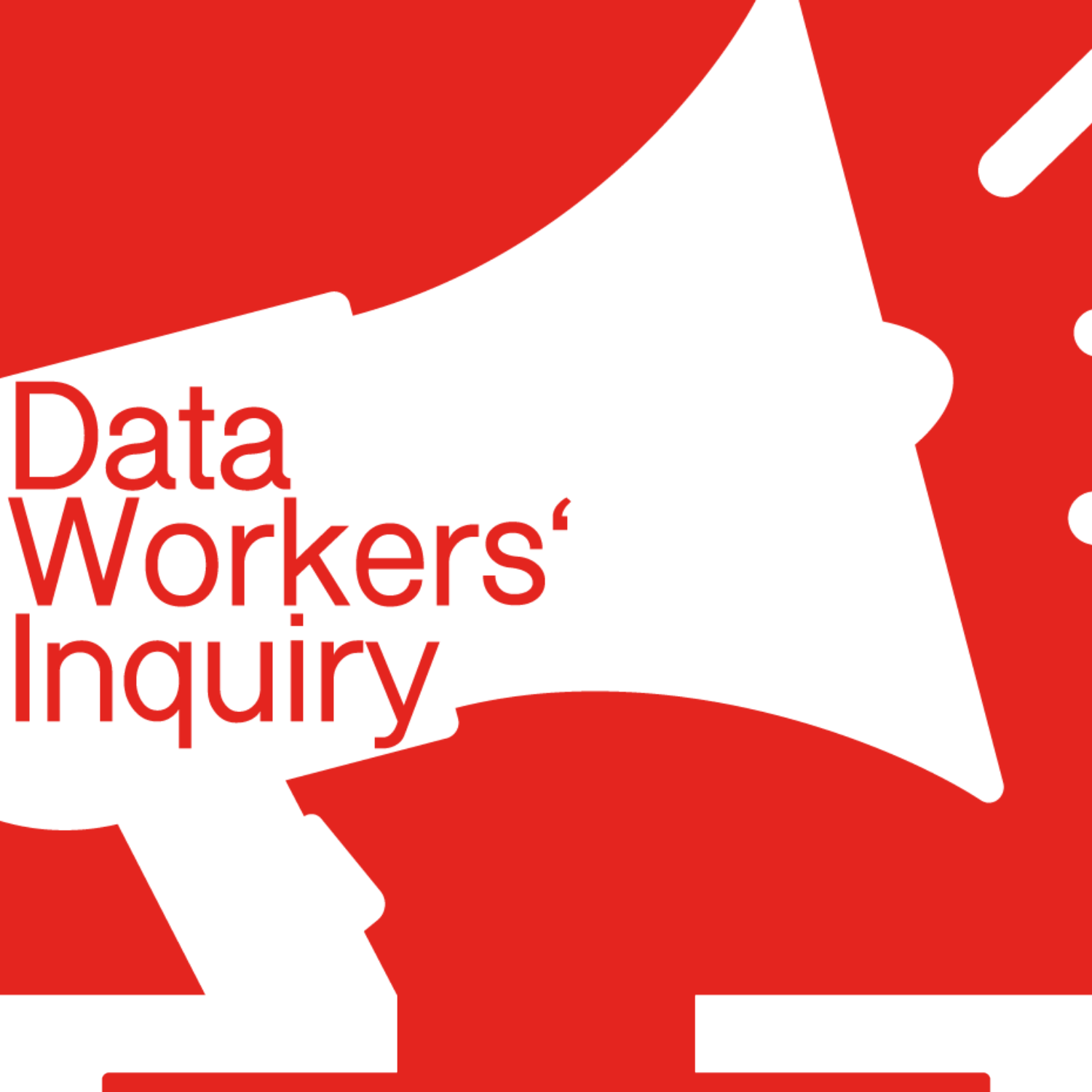
Data Workers Inquiry
15 data workers in Venezuela, Kenya, Syria, and Germany conduct research with their colleagues in their respective workplaces and reporting on labor conditions and widespread practices in the AI industry.
The Data Workers’ Inquiry is a community-based research project in which data workers join us as community researchers to lead their own inquiry in their respective workplaces. The community researchers guide the direction of the research, such that it is oriented towards their needs and goals of building workplace power but supported by formally trained qualitative researchers. We adapt Marx’s 1880 Workers’ Inquiry to the phenomenon of data workers who are both essential for contemporary AI applications yet precariously employed—if at all—and politically dispersed.
To explore the rest of the projects attached to the Data Workers' Inquiry, visit our website: data-workers.org/
35 playlists
Data Workers' Inquiry
15 data workers in Venezuela, Kenya, Syria, and Germany conduct research with their colleagues in their respective workplaces and reporting on labor conditions and widespread practices in the AI industry.
The Data Workers’ Inquiry is a community-based research project in which data workers join us as community researchers to lead their own inquiry in their respective workplaces. The community researchers guide the direction of the research, such that it is oriented towards their needs and goals of building workplace power but supported by formally trained qualitative researchers. We adapt Marx’s 1880 Workers’ Inquiry to the phenomenon of data workers who are both essential for contemporary AI applications yet precariously employed—if at all—and politically dispersed.
To explore the rest of the projects attached to the Data Workers' Inquiry, visit our website: data-workers.org/
Data Interception Environment Tutorial
Guide to using version 3 of PI's data inteception environment developed by me